Memory is what we are. Our memories shape us. Sometimes we remember. Other times we simply perceive differently. Why is it beneficial to have both explicit and implicit memories? Why do we find so many kinds of memory, and how to optimize the use of various memory kinds? Artificial intelligence training may provide a valuable insight into these processes.
AI training
Human memory is complex and hard to research. Artificial intelligence is almost fully controlled by its developers. Typically artificial intelligence is trained on some dataset, which is a sort of explicit memory. After training, the “lessons learned” are stored for example as weights of the neural network. This is a different kind of memory, I would like to call implicit.
When we examine the training and validation datasets we typically understand very well the data and its meaning. Examining the weights of the neural network is harder and inconclusive. More often than not we cannot explain the role of a specific weight function. There are some attempts to provide more transparency into neural networks and remove arbitrary weights. Occasionally such attempts provide valuable insights, but they are inconclusive.
In other places, I mention “know what” vs “know how”. Knowing factoids does not necessarily enable hands-on practical skills. Quite often when applying hands-on practical skills, we cannot explain how they work.
Subliminal memories
Suppose we train our artificial intelligence with two datasets: private and public. We might be able to provide others with public datasets, but private datasets are typically secret for the clients, and often secret for people training the artificial intelligence.
In this example, the private data might be decipherable, but we cannot or do not bother to address it.
In our personal memories, some subliminal memories are like that. If we could remember them we might understand them, but we lack some recall cues. We might be able to overcome this limitation in our sleep or under hypnosis.
Revisiting memories in our sleep
An AI model may train with new data to adapt and improve, but to ensure its stability we may also add some old records to the new inputs. This is especially true for rare poorly represented situations, which might not be covered by new data.
When we sleep, during REM stage, our brain often accesses explicit and subliminal memories, to improve the implicit models involved in decision-making and physical activities. We feel like we run through some sort of simulation. Our eyes move, and our brain waves are fast, processing new scenarios.
Episodic vs semantic memory
In artificial intelligence, we have supervised and unsupervised learning. When we deal with supervised learning, the data is labeled. Usually, this means that we have less data to work with, but learning is easier. In unsupervised learning, we usually have a lot of data but the data is not labeled.
In our own brains, we have semantic and episodic memory. Semantic memory typically deals with rules and labeled data, like words and math. Episodic memory usually deals with various events and anecdotes. These events and anecdotes are not explained, and each time we process them we may discover new things.
Many memories used by experts
The list of various kinds of memory used by experts is very long. Is this justified or artificial? Regular experiences fall somewhere between multiple kinds of memory, as we feel and hear, analyze and recall, feel and articulate – simultaneously. To get specific experiments that work with a specific kind of memory, experts design various games and experiments.
Experts need multiple highly differentiated kinds of memory to generate more scientific publications. The results will vary per memory kind when we address for example age and gender groups. This is an easy recipe to produce scientific papers.
AI experts need multiple types of memory to optimize hardware purchases and architecture. Some kinds of memory need to be faster or better connected than other kinds of memory. If we train a neural network, the network weights are always active, but the data in training datasets is addressed in small chunks sequentially. So it is reasonable to use different kinds of memory hardware.
New and old memories
As strange as it sounds, the biggest difference between memory types is old vs new memory. Some people with neurological damage simply cannot form new memories. Others may have issues with transitioning from short-term memories to long-term memories. We all know stories about old-timers who can remember every detail of their youth but have no idea what happened yesterday.
Strangely, neuroplasticity issues do not really exist in AI. An engineer may set up a learning rate so that the system stops forming new memories of forgets its old memories. This is something introduced by engineers, but not by hardware.
Real memory vs simulated data
It is very hard to distinguish between a memory of a real event and a memory of a simulated event like a vivid dream. The effect of REM dreaming on our processing and decision-making is not very different compared with real experiences. To make things worse we remember the events based on our most recent recall, rather than the original event. Typically to understand which version of events is real we need to analyze the chain of events before and after as well as details for discrepancies. Still, there are some events in my life that exist as memories of both kinds and I do not know which version really happened.
For AI the situation is even worse. Generative networks may often generate adversarial scenarios altering the decision by switching a couple of pixels in the image that is used as input for the neural network. As humans, we are not that easily fooled.
Spatial memory
Memory masters have a warm spot in their hearts for spatial memory. It is possibly the only kind of memory where males outperform females. Spatial memory is processing-intensive, and it is especially fast in young males. The vast majority of mnemonic techniques focus on encoding and placing effectively unstructured data from multiple sources into rigid spatial structures. Our human brain as a hardware prefers 2D processing. The neocortex is basically a multi-layer sheet of neural cells forcefully compacted into a 3D structure.
AI is also very well-architectured to process two-dimensional data. All kinds of information are often encoded into 2D blocks of the same size. While it is possible to work with 1D or 3D data, 2D data is usually more efficient to work with and has better hardware acceleration. As we add dimensions, we lose some transparency in the analysis. The network connectivity often increases, and there are fewer connections with zero contribution to the decision-making.
Can we understand a neural network?
Sufficiently large neural networks are not very transparent. We do not fully understand the role of a single weight of an AI system in its decision-making. Possibly such analysis is not well formulated as the system functions as a whole. Even when we know that the first three layers of the network roughly act as edge detectors, we may not understand the exact mechanics of the computation.
Understanding the human brain is even harder. A possible way of trying to understand various brain areas is by analyzing the brain activity when performing various tasks. By staging experiments focusing on very specific tasks, scientists may occasionally pinpoint the relevant areas in the brain. If the experiment is not very well stage, a lot of brain areas will light up as a Christmas tree.
An alternative approach is analyzing brain-damaged people, for example, those who suffered a very specific brain trauma. Some brain functions will work perfectly, while other brain functions will suffer. Then we can say which brain areas contribute to the specific functions. Does such analysis work? Sometimes. Human brains are highly adaptable, and various parts of the brain can often perform similar functions compensating for damaged areas.
Brain will always be a mystery
Due to the complexity and implicit nature of some brain activities, human brain is likely to remain a mystery. Moreover, a sufficiently complex AI is also likely to remain a mystery, more than its hardware and software. AI systems usually undergo semi-random training, and occasionally systems with the same hardware and software might develop very different patterns.
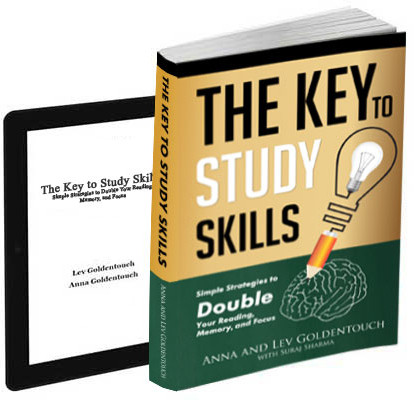
Get 4 Free Sample Chapters of the Key To Study Book
Get access to advanced training, and a selection of free apps to train your reading speed and visual memory