When looking for visual associations we can use the physical meaning of the concept. This method was so intuitive for me, that I never actually wrote about it. Only recently a student helped me figured out it is not used enough, and that very simple words are totally abstract for many students. I will use an actual communication with a real student of our 1:1 courses.
My field is too abstract for me
Hi Lev,
I am Henry, coming from Hong Kong and working as an equity analyst and I have already taken the become a super learner – the master class provided by Jonathan Levi before. I have learned and understood the fundamental elements of mnemonics and speed reading. Also, I can use the mnemonics to remember 20 random words and 20 random numbers within 1.5 minutes with high accuracy.
But most importantly, I find that my main problem is I do not know how to apply these skills in the reality, e.g. For my work, I need to read lots of financial news and financial reports. When I try to apply the skills on these reports and try to extract and remember those keywords, I find that it is very hard to remember the keywords as those keywords are quite technical and very abstract, e.g. very hard to change to markers and then remember effectively, not like the words I have seen in the exercises. So I would like someone to teach me how to apply these skills in reality step by step, remembering these technical keywords effectively and quickly.
Let take this article as an example.
Eight Predictions:
1. Productivity increases sharply. We quantified this in our July 2018 ‘Data Era’ report, suggesting that small improvements in corporate opex can lead to large gains across several industries: potentially equivalent to ~$3.6trn of gross savings, or 5% of global GDP. We believe that productivity gains will become more demonstrable. The industries that should benefit most are Process (Oil & Gas, Mining) and Healthcare.
2. Productivity tools get much better. As Adam Wood points out, too much technology investment in manufacturing today is really about retrofitting sensors and undertaking predictive maintenance. He sees greater value in combining PLM design and operation with the IoT hardware layer, i.e. properly integrating software. Additionally, the real benefits of cloud for manufacturing processes have not been tapped yet.
3. Efficiency becomes mandatory. If the introduction of basic monitoring software can reduce US power blackouts by 30% and save ~$23bn per annum, at what point does this become a consideration for regulators? Similarly, is the timely and accurate assessment of digital radiological information an ethical question? Similar considerations apply to the design of aircraft engines and deepwater oil facilities.
4. Manufacturing becomes customized – “Lot Size of One”. One of the benefits of virtualization, better design, and prototyping tools is customization: consumers are interested in ‘lot size of one’, and this can apply from trainers to phones to cars. Large-scale production of customized dental braces is another example.
5. Business models will change – but who keeps the spoils? In any technology shift, there is a natural tension between ‘enablers’ and ‘adopters’. In power generation (and potentially aircraft engines too), the introduction of sensors is pushing out scheduled service: that’s great news for utility customers, less so for power gen OEMs. Industrial enablers are pushing for revenue-sharing arrangements: it remains to be seen whether they are successful here.
6. Robotic automation will increase. With ongoing advances in machine vision and improvements in robotic dexterity, it seems inevitable that collaborative robots will gain share (they account for 3% of industrial robots today). At present, there are 85 robots for every 10,000 manufacturing employees or a penetration rate of 0.9%. Assuming a 10-15% CAGR (as per estimates from Mordor Intelligence), this could reasonably grow to 7% (low) or 18% (high) penetration by 2040.
7. An inverted computing world and the end of Moore’s Law. The last 70 years of exponential data growth has been fueled by the supply side of technology-driven by Moore’s Law – but the ability to run faster with more transistors per chip ended a decade ago. To continue advancing computing capabilities at reduced cost will require entirely new semiconductor process and device technology focused on demand. The required capital investment in next-generation factories will quickly exceed US$20bn in the coming 3-5 years.
8. Human-Machine interface becomes continuous. Recent advances in bionic robotics, neurology, and embedded sensors are leading to remote wireless control of machines for the first time. There has been significant progress since 2011 in the advancement of nano-sensors – for example, ‘neural dust’. It seems likely that there will be greater connectivity between human and artificial intelligence.
Here are my markers, productivity, tools, efficiency, customization, business models, robotics, human-machine interface.
However, I have some difficulties to create images for those abstract words including productivity, efficiency, customization, business models. So I cannot create the markers effectively and quickly.
Thank you!
Henry
Wait, this is very simple.
Dear Henry,
These are very easy to generate and then you reuse them. Try going for physical meaning. Consider apple juice production.
Sincerely,
Lev
OK, so what happened
While we offer some pretty unique and very helpful material in our keytostudy masterclasses, sometimes the students simply need 1:1 guidance to understand how these concepts apply in their particular case. There are several very common complaints: my materials are very complex or very abstract, it takes me forever to generate the relevant visualizations, I do not understand what to mark and visualize. These issues are common.
We advise (with more examples in my book) finding some simple example where all of the ideas work. I call it “the physical meaning”. Physicists often use simple mechanical metaphors to describe very complex intuition. For example, the way gravity influences space and time can be seen as a big ball pressing on a flexible rubber sheet.
When people create abstract concepts, they usually see several similar examples and give a name to the common factor. We can go the opposite way: take the abstract name and derive the examples where it was used. Engineers and physicists do this almost instinctively, but people working with money and marketing often struggle with it. Personally I developed the skill about a decade ago after reading this book.
Why our expertise is the hardest
Visualizing our own expertise is usually very hard because we drown in details. We know so much detailed information about every issue, that we find it hard to generalize and simplify. I personally struggle visualizing mathematical formulas. Everything else for me is very easy in comparison, even monstrous molecules of organic chemistry. At least those do not mix greek letters and scalable abstractions.
Everything from the world of finance, law, computers or engineering is based on real life examples. If we can find those examples, we will visualize easier and have a very deep intuition of our subject.
Consider this exercise: explain what you do at work to a smart 10 years old. If you are successful, reuse the metaphors you used for visualization. If you failed, enlist the help of your spouse.
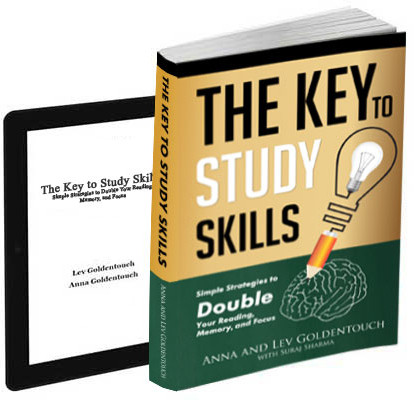
Get 4 Free Sample Chapters of the Key To Study Book
Get access to advanced training, and a selection of free apps to train your reading speed and visual memory